この記事は以下のサイトの翻訳記事です。
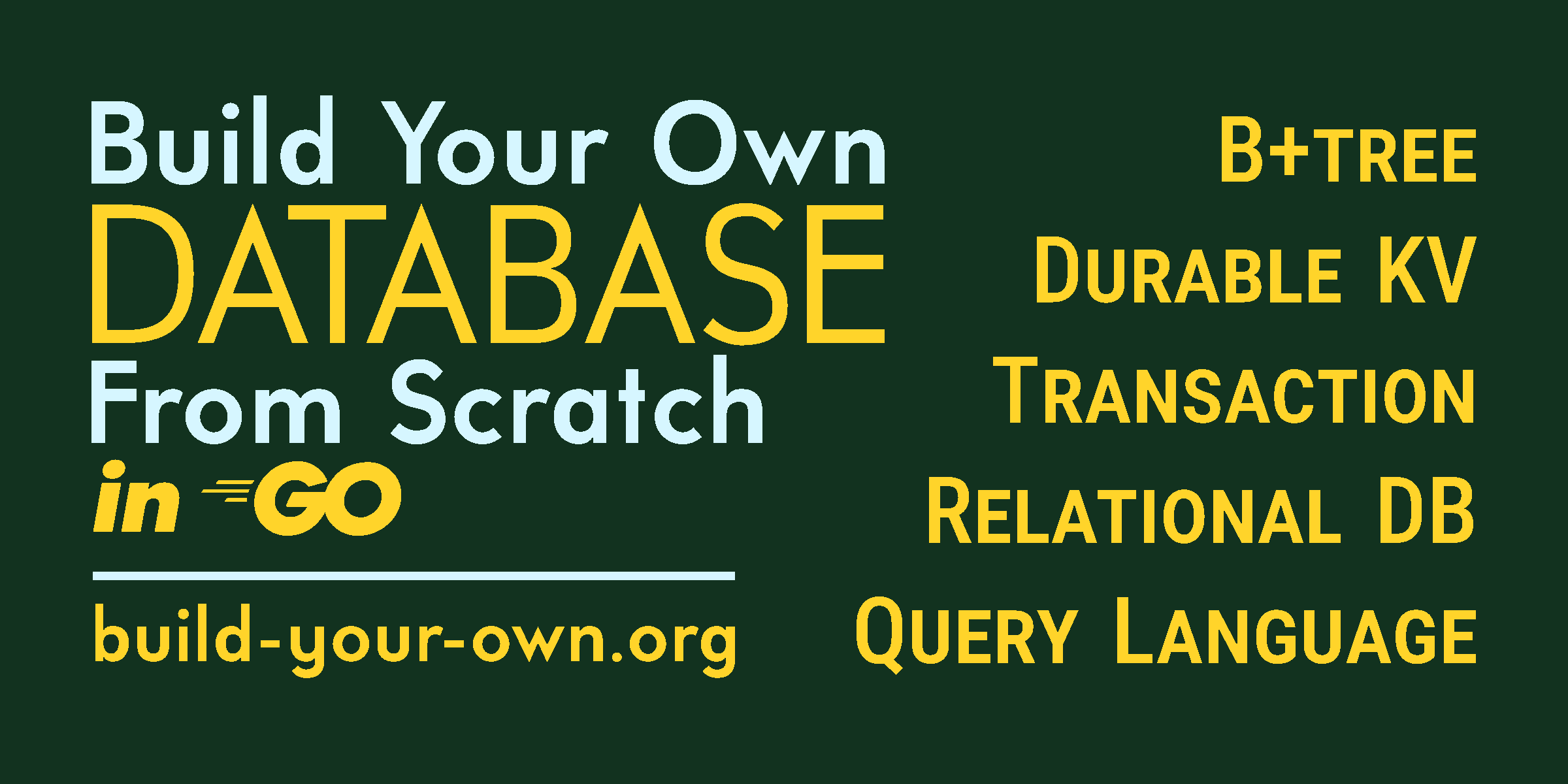
B-tree は平衡 n 分木である
平衡木(Height-balanced tree)
実用的な二分木の多く、たとえば AVL木 や 赤黒木(RB木) は「平衡木(height-balanced tree)」と呼ばれます。これは、木の高さ(ルートから葉まで)が O(log N) に抑えられていることを意味し、そのため 検索処理も O(log N) で行えます。
B-tree も平衡 n 分木であり、すべての葉ノードが同じ高さに位置しています。
Many practical binary trees, such as the AVL tree or the RB tree, are called height-balanced trees, meaning that the height of the tree (from root to leaves) is limited to O(log N), so a lookup is O(log N).
A B-tree is also height-balanced; the height is the same for all leaf nodes.
2分木の一般化
n 分木は二分木を一般化したもの(あるいはその逆)と考えることができます。たとえば 2-3-4 木 は、各ノードが 2、3、または 4 個の子ノードを持つことができる B-tree です。2-3-4 木は赤黒木(RB木)と等価な構造ですが、B-tree を理解する上では詳細を知る必要はないため、ここでは深入りしません。
ソート済みの列 [1, 2, 3, 4, 6, 9, 11, 12]
に対する 2 段構成の B+tree の例を視覚化すると以下のようになります:
[1, 4, 9]
/ | \
v v v
[1, 2, 3] [4, 6] [9, 11, 12]
B+tree では、値を保持するのは葉ノードのみであり、内部ノードには 部分木のキー範囲を示すためのキーが複製されます。
この例では、ノード [1, 4, 9]
は、3つの部分木がそれぞれ [1, 4)
, [4, 9)
, [9, +∞)
の範囲に対応していることを示しています。
ただし、3つの区間を表現するにはキーは2つだけで十分なので、先頭のキー(1)は省略可能です。
その場合、3つの区間は (-∞, 4)
, [4, 9)
, (9, +∞)
となります。
n-ary trees can be generalized from binary trees (and vice versa). An example is the 2-3-4 tree, which is a B-tree where each node can have either 2, 3, or 4 children. The 2-3-4 tree is equivalent to the RB tree. However, we won’t go into the details because they are not necessary for understanding B-trees.
Visualizing a 2-level B+tree of a sorted sequence [1, 2, 3, 4, 6, 9, 11, 12].
[1, 4, 9] / | \ v v v [1, 2, 3] [4, 6] [9, 11, 12]
In a B+tree, only leaf nodes contain value, keys are duplicated in internal nodes to indicate the key range of the subtree. In this example, node [1, 4, 9] indicates that its 3 subtrees are within intervals [1, 4), [4, 9), and [9, +∞). However, only 2 keys are needed for 3 intervals, so the first key (1) can be omitted and the 3 intervals become (-∞, 4), [4, 9), and (9, +∞).
B-tree をネストされた配列として捉える
2段階のネスト配列
RB木や2-3-4木の詳細を知らなくても、B-tree はソート済み配列の構造から理解することができます。
ソート済み配列の問題点は、更新コストが O(N) であることです。
この配列を 重なりのない小さな配列に m 個に分割すれば、更新コストは O(N/m) に抑えられます。
ただし、どの小さな配列を更新または検索すればよいかを判断する必要があるため、小さな配列への参照を持つもう1つのソート配列が必要になります。これが B+tree における内部ノードです。ソート済み配列の問題点は、更新コストが O(N) であることです。
この配列を 重なりのない小さな配列に m 個に分割すれば、更新コストは O(N/m) に抑えられます。
ただし、どの小さな配列を更新または検索すればよいかを判断する必要があるため、小さな配列への参照を持つもう1つのソート配列が必要になります。これが B+tree における内部ノードです。
[[1,2,3], [4,6], [9,11,12]]
この構成では、2 回の二分探索で O(log N) の探索が可能です。
もし m = √N
と設定すれば、更新コストは O(√N) になり、2段階のソート配列としては最良の性能になります
Without knowing the details of the RB tree or the 2-3-4 tree, the B-tree can be understood from sorted arrays.
The problem with sorted arrays is the O(N) update. If we split the array into m smaller non-overlapping ones, the update becomes O(N/m). But we have to find out which small array to update/query first. So we need another sorted array of references to smaller arrays, that’s the internal nodes in a B+tree.
[[1,2,3], [4,6], [9,11,12]]
The lookup cost is still O(log N) with 2 binary searches. If we choose m as √N, update become O(√N), that’s as good as 2-level sorted arrays can be.
多段階のネスト配列
O(√N) の更新コストはデータベース用途としては許容できません。
しかし、配列をさらに細かく分割して階層を増やすことで、コストをさらに削減することができます。
たとえば、すべての配列が 定数 s 以下のサイズになるまで階層的に分割していくと、最終的には log (N/s) 段の階層になります。
このときの検索コストはO(log (N/s) + log (s)) = O(log N) となり、依然として効率的です。
挿入や削除においては、目的の葉ノードを見つけた後、そのノードの更新は通常 O(s) の定数時間で済みます。
残された課題は、各ノードのサイズが s 以下であること、および 空にならないことという 不変条件(invariants)を保つことです。
O(√N) is unacceptable for databases, but if we add more levels by splitting arrays even more, the cost is further reduced.
Let’s say we keep splitting levels until all arrays are no larger than a constant s, we end up with log (N/s) levels, and the lookup cost is O(log (N/s) + log (s)), which is still O(log N).
For insertion and deletion, after finding the leaf node, updating the leaf node is constant O(s) most of the time. The remaining problem is to maintain the invariants that nodes are not larger than s and are not empty.
B+tree のメンテナンス
B+tree を更新する際に守るべき 3 つの不変条件(invariant):
- すべての葉ノードが同じ高さにあること
- ノードのサイズが定数で制限されていること
- ノードが空でないこと
3 invariants to preserve when updating a B+tree:
- Same height for all leaf nodes.
- Node size is bounded by a constant.
- Node is not empty.
ノードの分割による B-tree の成長
B-tree における第2の不変条件(ノードサイズの上限)は、葉ノードへの挿入によって破られることがあります。これを解消するために、ノードをより小さなノードに分割して構造を保ちます。
parent parent
/ | \ => / | | \
L1 L2 L6 L1 L3 L4 L6
* * *
葉ノードを分割すると、その親ノードに新たな枝(子ノードへの参照)が追加されます。
この結果、親ノードのサイズも上限を超える可能性があり、その場合は親ノードも分割が必要になります。
こうして分割処理がルートノードまで伝播すると、木全体の高さが1つ増加します。
new_root
/ \
root N1 N2
/ | \ => / | | \
L1 L2 L6 L1 L3 L4 L6
この操作によって、すべての葉ノードが同時に1段階高くなるため、第1の不変条件(葉ノードの高さが同じであること)も保たれます。
The 2nd invariant is violated by inserting into a leaf node, which is restored by splitting the node into smaller ones.
parent parent / | \ => / | | \ L1 L2 L6 L1 L3 L4 L6 * * *
After splitting a leaf node, its parent node gets a new branch, which may also exceed the size limit, so it may need to be split as well. Node splitting can propagate to the root node, increasing the height by 1.
new_root / \ root N1 N2 / | \ => / | | \ L1 L2 L6 L1 L3 L4 L6
This preserves the 1st invariant, since all leaves gain height by 1 simultaneously.
ノードのマージによる B-tree の縮小
削除操作によって、ノードが空になる場合があります。
このときは、空になったノードを兄弟ノードとマージすることで、第3の不変条件(ノードが空でないこと)を回復します。
マージは分割の逆の操作であり、ルートノードまで伝播する可能性があるため、木の高さが1つ下がることもあります。
B-tree を実装する際には、空になる前に早めにマージを行うことで、無駄なメモリを減らすこともできます。
たとえば、ノードのサイズが下限に達した段階でマージするという方法もあります。
Deleting may result in empty nodes. The 3rd invariant is restored by merging empty nodes into a sibling node. Merging is the opposite of splitting. It can also propagate to the root node, so the tree height can decrease.
When coding a B-tree, merging can be done earlier to reduce wasted space: you can merge a non-empty node when its size reaches a lower bound.
3.4 B-Tree on disk
これまでの原則を使えば、メモリ上の B-tree はすでに実装可能です。
しかし、ディスク上で B-tree を実装するには、追加の配慮が必要になります。
You can already code an in-memory B-tree using these principles. But B-tree on disk requires extra considerations.
ブロック単位の割り当て
ここまでの説明で触れられていない重要な点は、ノードサイズの制限方法です。
メモリ上の B+tree であれば、ノード内のキーの数に上限を設ければ十分であり、バイトサイズを気にする必要はありません。なぜなら必要なだけメモリ(バイト)を割り当てられるからです。
一方、ディスクベースのデータ構造では malloc/free やガーベジコレクタのような仕組みは存在せず、
領域の割り当てと再利用はすべて自分で管理する必要があります。
もしすべての割り当てサイズが同じであれば、空きリスト(free list) を使って領域の再利用を行うことができます。
これは後ほど実装しますが、今のところは、すべての B-tree ノードを同じサイズで扱うことにします。
One missing detail is how to limit node size. For in-memory B+tree, you can limit the maximum number of keys in a node, the node size in bytes is not a concern, because you can allocate as many bytes as needed.
For disk-based data structures, there are no
malloc/free
or garbage collectors to rely on; space allocation and reuse is entirely up to us.Space reuse can be done with a free list if all allocations are of the same size, which we’ll implement later. For now, all B-tree nodes are the same size.
安全な更新のための Copy-on-Write B-tree
これまでに、ディスク上のデータをクラッシュに強く更新する方法として、ファイルのリネーム、ログ、LSM-tree の3つを見てきました。
ここから得られる教訓は、更新中に既存のデータを破壊しないことです。
この考え方はツリー構造にも応用できます:ノードをコピーし、そのコピーを変更するのです。
挿入や削除は葉ノードから始まります。変更を加えたコピーを作ったあと、その親ノードも新しいノードを参照するように更新する必要があります。
この更新もコピー上で行われ、コピー操作はルートノードまで伝播します。
その結果、新しいルートを持つ B-tree が出来上がります。
元のツリーはそのまま保持されており、以前のルートからアクセス可能です。
新しいルートは、葉ノードまでのコピーされたノードを含みますが、それ以外のノードは元のツリーと共有しています。
d d D*
/ \ / \ / \
b e ==> b e + B* e
/ \ / \ / \
a c a c a C*
original updated
葉ノード c
を更新する際の視覚的なイメージです。
コピーされたノードは大文字(D, B, C)で、共有されている部分木は小文字(a, e)で表されています。
このような構造は コピー・オン・ライト(copy-on-write)データ構造と呼ばれます。
文献によっては、これを「イミュータブル(immutable)」「追記専用(append-only)※文字通りではない」「永続的(persistent)※永続化とは別概念」などと説明することもあります。
ただし、データベースの用語は一貫した意味で使われていないことがあるため注意が必要です。
Copy-on-Write B-tree に残る課題はあと2つあります:
- ツリーのルートをどう見つけるか?
更新のたびにルートが変わるため、クラッシュ耐性の問題は「ポインタ1つの更新」に帰着します。
この問題については後ほど解決します。 - 古いバージョンのノードをどう再利用するか?
これは フリーリスト(free list) の役割となります。
We’ve seen 3 crash-resistant ways to update disk data: renaming files, logs, LSM-trees. The lesson is not to destroy any old data during an update. This idea can be applied to trees: make a copy of the node and modify the copy instead.
Insertion or deletion starts at a leaf node; after making a copy with the modification, its parent node must be updated to point to the new node, which is also done on its copy. The copying propagates to the root node, resulting in a new tree root.
- The original tree remains intact and is accessible from the old root.
- The new root, with the updated copies all the way to the leaf, shares all other nodes with the original tree.
d d D* / \ / \ / \ b e ==> b e + B* e / \ / \ / \ a c a c a C* original updated
This is a visualization of updating the leaf c. The copied nodes are in uppercase (D, B, C), while the shared subtrees are in lowercase (a, e).
This is called a copy-on-write data structure. It’s also described as immutable, append-only (not literally), or persistent (not related to durability). Be aware that database jargon does not have consistent meanings.
2 more problems remain for the copy-on-write B-tree:
- How to find the tree root, as it changes after each update? The crash safety problem is reduced to a single pointer update, which we’ll solve later.
- How to reuse nodes from old versions? That’s the job of a free list.
Copy-on-Write B-tree の利点
古いバージョンを保持しておくことの利点の一つは、スナップショット分離(snapshot isolation)を無料で手に入れられることです。
トランザクションは特定のツリーバージョンから開始され、他のバージョンによる変更を一切見ることはありません。
また、クラッシュからの復旧も非常に簡単です。直前の古いバージョンを使えば良いだけです。
さらに、複数リーダー・単一ライター(multi-reader-single-writer)型の並行処理モデルに適しており、リーダーがライターをブロックしないという利点もあります。
これらについては、後ほど詳しく見ていきます。
One advantage of keeping old versions around is that we got snapshot isolation for free. A transaction starts with a version of the tree, and won’t see changes from other versions.
And crash recovery is effortless; just use the last old version.
Another one is that it fits the multi-reader-single-writer concurrency model, and readers do not block the writer. We’ll explore these later.
代替案:ダブルライトによるインプレース更新
Copy-on-Write データ構造ではクラッシュ復旧が明確である一方で、書き込み増幅が大きくなるというデメリットがあります。
各更新で経路全体をコピーする必要があり(O(log N))、一方で インプレース更新なら通常は1つの葉ノードだけを書き換えるだけで済むことが多いのです。
Copy-on-Write を使わなくても、クラッシュ復旧可能なインプレース更新を行うことは可能です:
- 更新対象のノード全体をどこかに保存しておく(これは Copy-on-Write に似ているが、親ノードはコピーしない)
- 保存したコピーに対して
fsync
を行う(このタイミングでクライアントに応答可能) - 実際に データ構造をインプレースで更新する
- 更新後のデータに対して
fsync
を行う
クラッシュ後は、データ構造が中途半端に更新された状態かもしれませんが、それを判別する必要はありません。
とにかく保存しておいたコピーを無条件で適用することで、常に「更新済みの状態」に持っていくことができます。
| a=1 b=2 |
|| 1. Save a copy of the entire updated nodes.
\/
| a=1 b=2 | + | a=2 b=4 |
data updated copy
|| 2. fsync the saved copies.
\/
| a=1 b=2 | + | a=2 b=4 |
data updated copy (fsync'ed)
|| 3. Update the data structure in-place. But we crashed here!
\/
| ??????? | + | a=2 b=4 |
data (bad) updated copy (good)
|| Recovery: apply the saved copy.
\/
| a=2 b=4 | + | a=2 b=4 |
data (new) useless now
MySQL の用語では、保存しておいた更新済みのコピーのことを ダブルライト(double-write) と呼びます。
では、もしそのダブルライト自体が壊れていたらどうなるでしょうか?
この場合もログと同様に、チェックサムで対応します。
- チェックサムでダブルライトの破損が検出された場合:それを無視します。
これは 最初のfsync
の前の状態なので、メインのデータはまだ「古くて正しい状態」にあります。 - ダブルライトが正常だった場合:それを適用すれば、必ず メインデータは正しい状態になります。
一部のデータベースでは、ダブルライトをログの中に保存しています。これを 物理ログ(physical logging) と呼びます。
ログには2種類あり:
- 論理ログ(logical logging):
「キーの挿入」などの高レベルな操作を記述します。
これは データベースが正常な状態でないと適用できません。 - 物理ログ(physical logging):
ディスクページのような低レベルな更新内容を記録します。
このため、障害からの復旧には物理ログの方が有用なのです。
While crash recovery is obvious in copy-on-write data structures, they can be undesirable due to the high write amplification. Each update copies the whole path (O(log N)), while most in-place updates touch only 1 leaf node.
It’s possible to do in-place updates with crash recovery without copy-on-write:
- Save a copy of the entire updated nodes somewhere. This is like copy-on-write, but without copying the parent node.
fsync
the saved copies. (Can respond to the client at this point.)- Actually update the data structure in-place.
fsync
the updates.After a crash, the data structure may be half updated, but we don’t really know. What we do is blindly apply the saved copies, so that the data structure ends with the updated state, regardless of the current state.
| a=1 b=2 | || 1. Save a copy of the entire updated nodes. \/ | a=1 b=2 | + | a=2 b=4 | data updated copy || 2. fsync the saved copies. \/ | a=1 b=2 | + | a=2 b=4 | data updated copy (fsync'ed) || 3. Update the data structure in-place. But we crashed here! \/ | ??????? | + | a=2 b=4 | data (bad) updated copy (good) || Recovery: apply the saved copy. \/ | a=2 b=4 | + | a=2 b=4 | data (new) useless now
The saved updated copies are called double-write in MySQL jargon. But what if the double-write is corrupted? It’s handled the same way as logs: checksum.
- If the checksum detects a bad double-write, ignore it. It’s before the 1st
fsync
, so the main data is in a good and old state.- If the double-write is good, applying it will always yield good main data.
Some DBs actually store the double-writes in logs, called physical logging. There are 2 kinds of logging: logical and physical. Logical logging describes high-level operations such as inserting a key, such operations can only be applied to the DB when it’s in a good state, so only physical logging (low-level disk page updates) is useful for recovery.
クラッシュ復旧の原則
ダブルライトとコピー・オン・ライトを比較してみましょう。
- ダブルライトは、更新を冪等(べきとう)にします。すなわち、保存したコピー(完全なノード)を適用することで、DB は更新を再試行できます。
- 一方、コピー・オン・ライトは、すべてを新しいバージョンに原子的に切り替えます。
これらは異なる考え方に基づいています:
- ダブルライトは、新しいバージョンを生成するために十分な情報を確保します。
- コピー・オン・ライトは、古いバージョンが保存されていることを保証します。
もし、ダブルライトで更新済みのノードではなく、元の(オリジナルの)ノードを保存したらどうなるでしょうか?
それは、コピー・オン・ライトのように古いバージョンに復旧する第3の方法となります。
これら3つの方法を1つの考え方にまとめると、「任意の時点で、古い状態あるいは新しい状態のいずれかを再現するのに十分な情報が存在している」ということになります。
また、どちらの場合も、ある程度のコピー処理は必ず必要となるため、ツリーノードが大きくなればなるほど更新が遅くなります。
今回はシンプルさのためにコピー・オン・ライトを採用しますが、ここで別の手法を採用しても構いません。
訳者の補足【Copy-on-WriteとDouble-writeの違い】
端的に書くとデータ更新前にコピーするか(Copy-on-write)、データ更新の際にコピーするのか(Double-write)の違いです
Copy-on-write は、更新前の状態をコピーし、そのコピーに対して変更を加えることで新しいバージョンを生成します。
一方、double-write は、更新後のデータを別の領域に書き出しておき、クラッシュ時にその完全な更新済みデータを使って復旧する方式です。
Let’s compare double-write with copy-on-write:
- Double-write makes updates idempotent; the DB can retry the update by applying the saved copies since they are full nodes.
- Copy-on-write atomically switches everything to the new version.
They are based on different ideas:
- Double-write ensures enough information to produce the new version.
- Copy-on-write ensures that the old version is preserved.
What if we save the original nodes instead of the updated nodes with double-write? That’s the 3rd way to recover from corruption, and it recovers to the old version like copy-on-write. We can combine the 3 ways into 1 idea: there is enough information for either the old state or the new state at any point.
Also, some copying is always required, so larger tree nodes are slower to update.
We’ll use copy-on-write because it’s simpler, but you can deviate here.
学んだこと
B+tree の原則:
- n 分木、ノードのサイズは定数で制限される
- すべての葉が同じ高さであること
- 挿入と削除のための分割とマージ
ディスクベースのデータ構造:
- Copy-on-write データ構造
- ダブルライトによるクラッシュ復旧
これでコーディングを始めることができます。
B+tree をベースにした永続的なキー・バリュー・ストアを作るための 3 つのステップ:
- B+tree のデータ構造を実装する
- B+tree をディスク上に移行する
- フリーリストを追加する
B+tree principles:
- n-ary tree, node size is limited by a constant.
- Same height for all leaves.
- Split and merge for insertion and deletion.
Disk-based data structures:
- Copy-on-write data structures.
- Crash recovery with double-write.
We can start coding now. 3 steps to create a persistent KV based on B+tree:
- Code the B+tree data structure.
- Move the B+tree to disk.
- Add a free list.